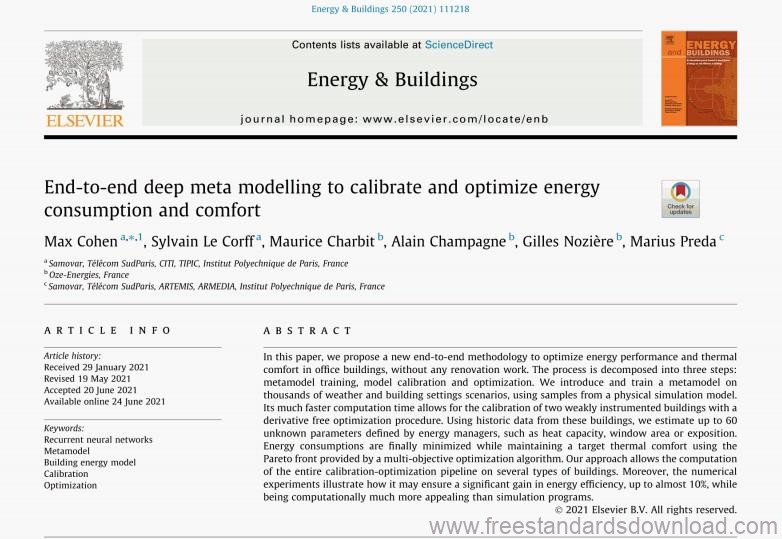
ABSTRACT
In this paper,we propose a new end-to-end methodology to optimize energy performance and thermal comfort in office buildings,without any renovation work.The process is decomposed into three steps:meta model training,model calibration and optimization.We introduce and train a meta model on thousands of weather and building settings scenarios,using samples from a physical simulation model.Its much faster computation time allows for the calibration of two weakly instrumented buildings with aderivative free optimization procedure.Using historic data from these buildings,we estimate up to 60 unknown parameters defined by energy managers,such as heat capacity,window area or exposition.Energy consumptions are finally minimized while maintaining a target thermal comfort using the Pareto front provided by a multi-objective optimization algorithm.Our approach allows the computation of the entire calibration-optimization pipeline on several types of buildings.Moreover,the numerical experiments illustrate how it may ensure a significant gain in energy efficiency,up to almost 10%,while being computationally much more appealing than simulation programs.